Generative AI Fundamentals: What Businesses Need To Know
- Lee Gang
- Sep 18, 2023
- 11 min read
Updated: Oct 10, 2023
Generative AI represents a transformative tool that businesses can utilize to streamline operations and foster innovation. But like any tool, knowing its capabilities and limitations is key to leveraging its full potential.
Summary
Generative AI should be treated as another tool that businesses can leverage to transform their businesses. Like any other tool, understanding how Generative AI works, what are its strengths and weaknesses are crucial to getting the best value out of Generative AI.
Generative models for text and images consist of three core components: the Foundation Model that processes and "understands" raw data, the Fine-Tuned Layer that specializes the model for specific tasks, and the Application Layer that provides a user interface for interaction.
Gen AI offers extensive benefits such as automating tedious tasks, enhancing personalization, accelerating R&D, fostering creativity, and improving resource efficiency, and scalability. It is especially useful for tasks requiring high degrees of human interaction, natural (human) language and image processing.
While powerful, Gen AI has limitations including speed, misinformation, reproducibility, and customizability. It also poses societal risks like data privacy concerns, ethical dilemmas, resource intensiveness, depersonalization, job displacement, and potential for misuse, along with regulatory challenges.
A simple step-by-step guide is proposed for businesses to identify and prioritize Gen AI use cases by evaluating their impact on business objectives and calculating ROI, considering both the capabilities and limitations of Gen AI.
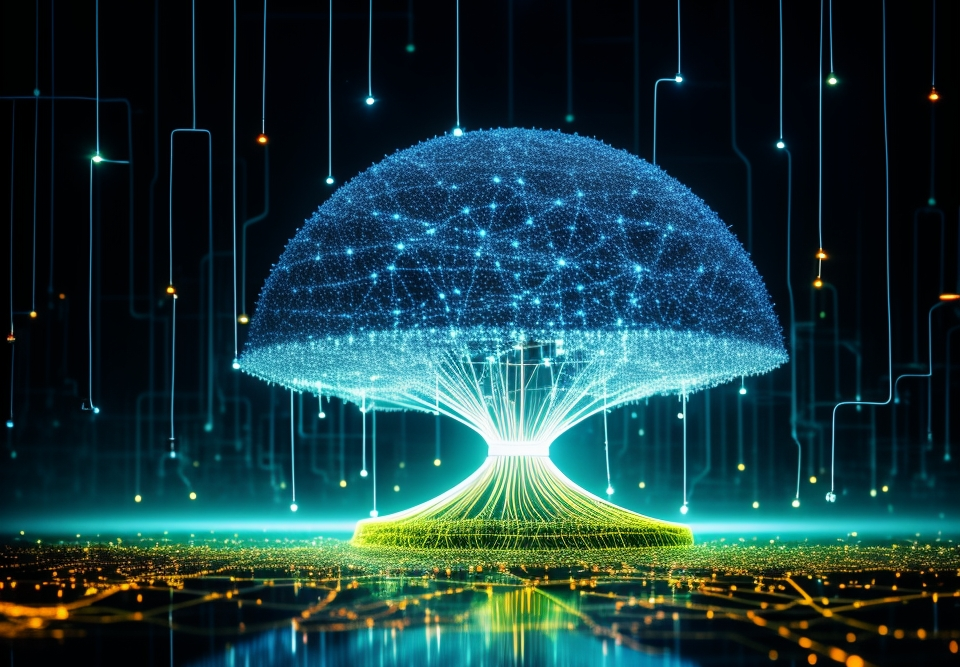
The digital landscape is flooded with news and developments about Generative AI (Gen AI). For business executives, keeping pace with these advancements can be overwhelming. With major enterprises making significant investments, such as EY’s $1.4 billion and AWS’ $100 million, it's easy to feel the FOMO pressure to jump onto the Gen AI bandwagon. What I hope to achieve in this article is to sift through the noise and distill what's truly important for businesses to know about Gen AI, while sharing some expert insights.
Understanding Generative AI
You're likely familiar with Gen AI and its applications like ChatGPT, Bard, Stable Diffusion, and Midjourney. Gen AI is a class of artificial intelligence models designed to generate new content based on the data they've been trained on. These models can produce a wide array of outputs, such as text, images, and even music, making them highly versatile in various applications. It differs from traditional or "discriminative" AI, which is more deterministic in nature. For a comprehensive yet non-technical explanation, I recommend this article from Gartner.
Generative AI is Just Another Tool
Like all technologies before it, Gen AI is just a tool. Despite all the hype, it is not a magical solution that makes your business problems go away. Like all tools, proper understanding of how it works, when it should be used, and when it shouldn’t is crucial in making the best use of it. Like a chainsaw, it's fantastic for some jobs but wholly unsuited for others. For the sake of conciseness, I will only focus on Gen AI that outputs text and image in this article. So, let’s delve into the workings of Gen AI.
Generative Text Models
Large Language Models (LLMs) are the linchpin of Gen AI's text-based applications. These models comprise three layers: the Foundation Model, the Fine-Tuned Layer, and the Application Layer.
Foundation Model: This is the initial layer that is developed using tons of text data from the internet and various other sources, and it is used to convert words, sentences and paragraphs of text into “embeddings”, which can be thought of as a series of numbers that the model uses to store the context and meaning of those words. Simply put, the Foundation Model translates human language in text into model language in a series of numbers that allows the model to “understand” human language. This can be thought of as the engine in cars, where it transforms chemical energy from fuel to mechanical energy. The Foundation Model requires the most resources to build compared to other components (foundation models can costs from a few million dollars to hundreds of million of dollars to train depend on size, some examples mentioned in this article). The capability of the foundation models to “understand” human language largely depends on its size, with popular foundation models ranging from 7B (7 billion model parameters) to 175B and more (for reference, GPT-4 is a combination of 8 x 220B models).
Fine-Tuned Layer: This layer refines the model for specialized tasks like question answering or code generation. It's akin to the vehicle’s wheels and drive shaft, transforming the engine's capabilities into directed movement. Different tasks are like connecting different components to the engine, some may use the engine to power large industrial machines, some to drive cars, others to power planes. The Fine-Tuned Layer is developed by providing it examples of data that it is expected to process, along with the corresponding expected output. For example, OpenAI's GPT-3.5-Turbo model has been fine-tuned for a human-like chat experience using a large set of questions and answers laboriously labelled by humans.
Application Layer: This is the user interface, allowing direct interaction with the model. For instance, ChatGPT's UI is an Application Layer that connects to the underlying model. The Application Layer can have other features built in as well, such as chat history and user authentication for ChatGPT. This is similar to the entire car, while containing the engine and drive shaft, also have other features such as seats, wind shields, radio etc. Different applications can be built on top of the model to cater to specific users and needs.
Generative Image Models
Generative image models are designed to produce visual content. Like their text-based counterparts, these models generally consist of three core layers:
Foundation Model: Pre-trained on a massive amount of visual data, ranging from photographs and paintings to diagrams and sketches. This initial layer translates pixels into a latent space—a numerical representation that captures the essential features of the images. These foundation models are also resource-intensive, with some requiring vast computational power and extensive data sets to train effectively.
Fine-Tuned Layer: This layer is trained on a narrower dataset to specialize in specific tasks like facial recognition, art generation, or medical imaging. For instance, a model might be fine-tuned to generate high-resolution artwork, while another might be calibrated for generating realistic terrains for video games.
Application Layer: This layer serves as the user interface for interacting with these image models. Applications may vary from simple image generators to complex design software suites, encapsulating the foundational and fine-tuned capabilities into a user-friendly package.
Just as in the text-based domain, generative image models can be modular. Some applications may solely use the Foundation Model for broad image recognition tasks, while others may incorporate Fine-Tuned Layers for specific industry applications, such as diagnostic medical imaging or architectural design.
Benefits and Advantages of Gen AI
According to a survey by Gartner, most companies focuses their Gen AI initiatives on customer experience / retention initiatives, followed by revenue growth and cost optimizations.

Below are some of the common benefits of Gen AI that businesses can utilize for their businesses:
Automating Tedious Tasks: One of the most immediately recognizable benefits of Gen AI is the automation of mundane, repetitive tasks. In the corporate world, for example, chatbots can handle customer service inquiries, freeing human operators to tackle more complex issues. In the medical field, image models can sift through X-rays or MRI scans to highlight potential areas of concern, augmenting the abilities of medical professionals. An example would be Amazon using Gen AI to summarize product reviews for customers.
Enhanced Personalization: Gen AI can be fine-tuned to understand and respond to individual user preferences. Whether it's a music recommendation engine or an online shopping assistant, these models can offer a highly personalized experience, leading to increased user engagement and satisfaction.
Accelerating Research and Development: Gen AI can rapidly generate and test multiple hypotheses or designs, thereby expediting the R&D process. In pharmaceuticals, for instance, AI can predict how different chemical compounds might behave, significantly shortening the drug discovery timeline. Gen AI also can augment or synthesize data, allowing organizations that lack their own data to test and develop new products using the synthesized data. For an example, Google is testing a product that uses generative AI technology to produce news stories and pitching it to news organizations.
Creativity and Innovation: The generative capabilities of these models extend to creative fields as well. From generating art and music to brainstorming product names and marketing strategies, Gen AI can be a valuable tool for enhancing human creativity. Gen AI are also used in the gaming industry to generate dynamic and new content.
Resource Efficiency: Despite the significant computational resources needed for training, once deployed, many Gen AI models can operate quite efficiently. This makes them cost-effective solutions for many applications, particularly when they can outperform or augment human capabilities.
Accessibility: Gen AI can also play a critical role in making technology more accessible. Text-to-speech and speech-to-text functionalities, powered by Gen AI, can make digital platforms more inclusive for people with disabilities.
Scalability: As AI models continue to improve, they offer the capability to scale services without a proportional increase in costs. This is particularly valuable for businesses looking to expand their operations or services without a linear increase in overheads.
Adaptability: The modular nature of Gen AI components—Foundation Model, Fine-Tuned Layer, and Application Layer—means that these systems can be adapted for a wide variety of applications. This flexibility allows organizations to pivot quickly in response to market changes or emerging opportunities.
To sum up, Gen AI is particularly useful in handling tasks that were previously hard to manage via traditional systems, such as tasks that involve high degree of human interaction, natural (human) language processing, and content generation and augmentation. It is touted as revolutionary as it is able to now solve problems which are previously almost impossible to solve automatically.
Risks and Disadvantages of Gen AI
While Gen AI offers a plethora of benefits, it is not a panacea to all problems. Specifically trained Traditional AI models still outperforms Gen AI in many supervised machine learning using (predictive) tasks using structured data. Optimization and mathematically complex tasks are still better handled through specific algorithms and formulas. Many also argued that human-generated creative content are still better than Gen AI generated contents. There are also a few key inherent disadvantages that comes with Gen AI (which may be resolved in the near future):
Speed: By nature of the large models used, processing an input and providing an output will take the model some time to process. This is usually longer for image or video format outputs and less so for text. Larger models also generally takes longer with some exceptions based on architectural differences. This is further exacerbated if the usage volume is high. The only ways to improve the processing speed is to invest in more computing resources (either more computers to cater to higher volumes, or increasing the capabilities of the computers to improve on individual response time, or both), and the shrink the model size (which may compromise on quality and accuracy). This limits the use of Gen AI in use cases where individual response speed is critical (e.g. high-frequency trading, emergency response coordination, autonomous vehicle navigation etc.).
Misinformation: Gen AI generated contents may not always be accurate. Some people called it “hallucinations”, where the model generates false information and state it as a fact. While there are some techniques that can reduce or prevent Gen AI providing false information, these techniques also limits the use cases where Gen AI can apply effectively to.
Reproducibility: Outputs generated by Gen AI models are not fully reproducible. Given the same inputs, Gen AI models might provide a different output which might be problematic for certain use cases where reproducibility of the output is critical (e.g. medical diagnosis and recommendation, automated grading etc.).
Customizability: Gen AI models are very difficult to customize. Organizations typically make changes to the Application Layer first due to the lower efforts required, followed by the Fine-Tuned Layer, which requires substantially more resources, not just data, but the expertise to fine tune the model. Making changes to these two layers might also be insufficient to to reach the minimum performance level required of high accuracy systems.
It is also crucial to consider the accompanying risks that come with its widespread adoption. Here are some of the main concerns:
Data Privacy and Security: Since Gen AI often requires large datasets to function effectively, there's an inherent risk related to the collection and storage of sensitive information. Data breaches could expose private information, posing significant risks to individuals and organizations.
Ethical Dilemmas: The use of AI in decision-making processes can lead to ethical concerns. For example, when employed in law enforcement or healthcare, an AI model that has been trained on biased data can perpetuate or even exacerbate existing societal inequalities.
Resource Intensiveness: Training advanced Gen AI models demands significant computational power, leading to high electricity consumption and thus, environmental concerns. The cost of maintaining and updating these models can also be prohibitive for smaller organizations.
Depersonalization: While AI can handle tasks traditionally done by humans, there's a risk of losing the human touch in services such as healthcare or customer service, where empathy and understanding are often crucial.
Job Displacement: Automation through Gen AI has the potential to displace workers in various sectors, from manufacturing to customer service. Although AI can create new job opportunities, the transition can be difficult for those who find their skills are no longer in demand.
Quality and Accuracy: Though AI has come a long way, the generated content is not always 100% accurate or reliable, which could be problematic in fields where precision is crucial, such as medical diagnosis or legal advice.
Potential for Misuse: The generative capabilities of AI can be used for unethical or illegal activities, such as deep fake creation, automated trolling, scam operations, or generating fake news. This could have serious social, political, and psychological ramifications.
Complexity and Unpredictability: Gen AI models, particularly huge models, are exceedingly complex. This complexity makes them difficult to interpret and understand, raising concerns about accountability and transparency in AI decision-making.
Regulatory Hurdles: As AI becomes more integrated into daily life, governments are already imposing regulations (e.g. EU AI Act) that could hinder the speed of development or limit the applications for which AI can be used, affecting the return on investment for companies in this space.
What’s It In For Businesses?
Now with a good understanding of how Gen AI works and what are its strengths and weaknesses, you can now look at your business to identify suitable use cases that can benefit from this tool. Below is a simplified step-by-step guide to help you identify potential use cases:
Catalog Business Processes: Start with listing all business processes in your business.
Identify Relevant Processes: Identify the processes and steps that involves any processing or generation of:
Queries, feedback, and engagement with customers, employees, or the public;
Text and document data;
Images, video or audio; and
Creative or professional content.
Spot New Processes: Identify the processes that you previously did not implement due to certain difficulty in processing or generating any of the information listed in (2).
Prioritize Processes Based On Business Impact: Evaluate the processes you've identified to determine which have the highest impact on your business objectives, be it revenue generation, customer satisfaction, or operational efficiency. Assign a priority level to each.
Match Capabilities: For each high-impact process, match it with the specific task to be performed by Gen AI — whether it's text generation, image recognition, or data analysis. This will give you an idea of which type of Gen AI model would be the most suitable and whether it is too complicated or infeasible altogether. Remove processes which are deemed unsuitable based on the risks and disadvantages of Gen AI mentioned above.
Assess Value: List down how each selected process will be like after incorporating Gen AI. Get an estimate of the value generated. List down both tangible (e.g. time savings, increased revenue, lower costs etc.) and intangible (e.g. access to new markets/audience, less conflicts among employees, improved corporate branding etc.) values. Try to convert the values into standard units for comparison and ROI calculation (typically in $).
Assess Effort: Assess how easily you could integrate Gen AI into each process. Consider factors like solution availability, data availability, required expertise, and cost. Also consider the time required till the solution is up and generating value (Time to Value).
Calculate ROI: Calculate the ROI for each selected process. Remove processes with no significant ROI. Rank the remaining based on each ROI.
With the above, you will have a list of potential use cases prioritized by their ROI for your business. However, there are a few more steps such as risk assessment, detailed feasibility study, and stakeholder engagement before moving on to implementation, topics I will delve into in future articles. Hopefully this article provided some conceptual understanding of Gen AI and provided some insights in generating value from it for your business. So what does your list of potential use cases look like? Feel free to share and discuss it in the comments.
Comments